Brains-py: The framework supporting HYBRAIN’s research on energy-efficient hardware
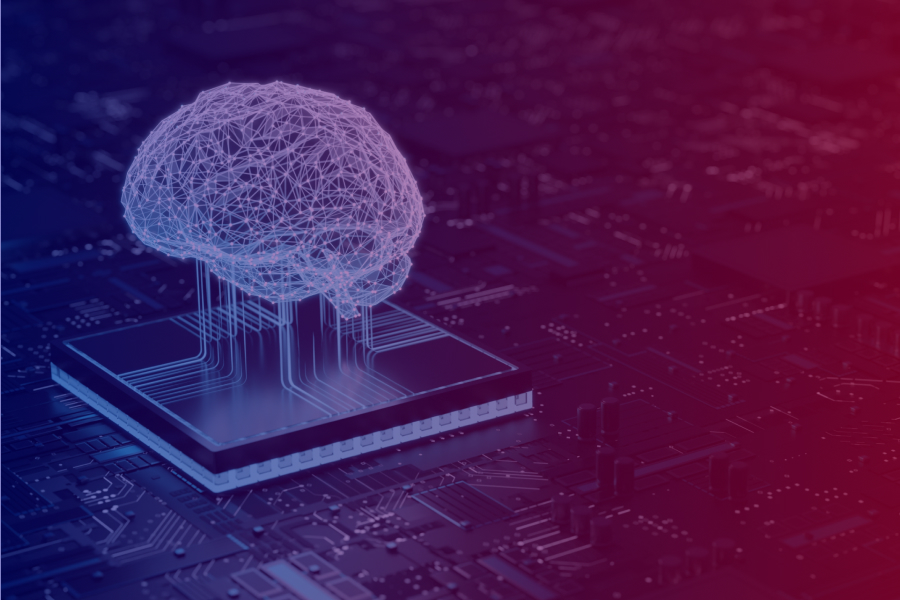
Brains-py is a versatile Python framework designed to support research on unconventional, energy-efficient hardware for machine learning. It particularly focuses on the utilisation and training of dopant network processing units (DNPUs), which are promising for their low-power consumption and CMOS compatibility.
Brains-py is crucial for the AI domain as it addresses the high energy costs associated with deep learning models. By enabling the research and development of DNPUs, this framework supports the creation of more sustainable and efficient AI hardware, potentially transforming AI infrastructure towards greater energy efficiency.
This framework facilitates significant advancements in the field of energy-efficient hardware for deep learning as it allows for the rapid exploration and scalability of DNPUs, supporting various supervised learning tasks through two main training approaches: evolution-in-matter and surrogate models. This dual approach aids in quick prototyping and effective scaling of DNPU-based circuits, proving essential for advancing machine learning hardware capabilities.
Importantly, the framework’s design ensures that simulations can be easily validated on hardware, enhancing the reproducibility and efficiency of research.
Brains-py and HYBRAIN
The development of Brains-py, which plays a pivotal role in advancing HYBRAIN’s goals, significantly benefits from the leadership of Wilfred van der Wiel, the coordinator of the HYBRAIN project. Van der Wiel’s role ensures that the insights and advancements in dopant network processing units (DNPUs) not only push the boundaries of machine learning hardware but also contribute directly to the goals of HYBRAIN, fostering a synergy between cutting-edge research and practical application in brain-inspired computing systems.